Is On-Premises Best for Advanced AI in the GenAI Maturity Model?

As generative AI (GenAI) redefines possibilities across industries, everyone is asking how organizations can measure their AI readiness and keep pushing capabilities further.
The GenAI Maturity Model offers a strategic path forward, guiding businesses from basic AI adoption to truly transformative, enterprise-level AI deployment. As companies reach higher levels of AI maturity, the need for on-premises solutions becomes a best practice, particularly for sophisticated players. Why?
Advanced GenAI deployments require unparalleled control over data, heightened security, and optimized performance—all of which on-prem solutions provide.
Let’s explore each level of the GenAI Maturity Model, offering insights into how businesses can progress through these stages, the strategic value of on-prem AI deployments, and how aligning AI initiatives with organizational goals drives sustainable success.
The GenAI Maturity Model: A Path to Strategic Growth and Operational Efficiency
The Generative AI (GenAI) Maturity Model is a comprehensive roadmap that guides organizations in strategically advancing their AI capabilities.
By understanding and progressing through each level, companies can move from initial exploration to fully integrated, enterprise-wide AI systems that generate real business impact.
In an era where AI applications are increasingly central to business operations, clarity on each level's goals, requirements, and expected outcomes is essential.
Level 1: No GenAI Strategy
At this foundational level, organizations haven’t established structured GenAI initiatives, and efforts are often limited to basic AI or automated tools applied sporadically across tasks. These companies rely on traditional decision-making approaches, and AI awareness is minimal.
To progress from this stage, organizations can start by educating key decision-makers on GenAI’s potential, perhaps through industry conferences, workshops, or expert-led sessions that build a foundational understanding of AI capabilities.
Level 2: GenAI Exploration
At this stage, organizations recognize GenAI's potential and explore its applications through small-scale initiatives or proofs of concept (POCs).
Key actions at this level include researching industry-specific applications and conducting trials with AI tools relevant to business needs, such as customer service or marketing. Early successes at this stage can help justify budget allocations for further exploration.
To advance, companies should identify business challenges where GenAI can offer real solutions, create cross-functional teams, and consider initial partnerships with AI vendors or consultants.
Level 3: Limited GenAI Adoption
In this phase, organizations start deploying GenAI within select business processes, moving beyond isolated trials to applications with measurable outcomes.
GenAI becomes a component of specific tasks, such as customer analytics or content generation, with initial returns on investment supporting further integration.
Progressing to the next level requires organizations to broaden GenAI adoption across different departments, align efforts with overarching business objectives, and ensure that ethical guidelines and data governance practices are in place.
Level 4: Focused GenAI Implementation
At Level 4, organizations develop a strategic approach to GenAI adoption, integrating it within their core operations.
Many establish dedicated GenAI teams or centers of excellence to manage deployment and align projects with larger organizational goals.
Data governance and ethical use guidelines are formalized, and investments in advanced tools are prioritized to support ongoing GenAI projects.
To continue maturing, organizations can focus on scaling successful GenAI applications across departments, driving higher returns on investment, and exploring options for developing custom models tailored to their unique business needs.
Level 5: Scaled GenAI Integration
At this level, organizations use GenAI widely across multiple functions and departments, making it an integral part of operations.
Customized AI models are developed to address specific needs and are supported by robust data infrastructure and efficient pipelines that sustain large-scale GenAI initiatives.
Decision-making is increasingly AI-driven, and continuous training programs ensure employees stay current with AI technologies.
Moving forward, these companies can explore industry-specific AI applications that deliver strategic insights, develop AI-driven products or services, and establish internal data architecture for advanced AI applications.
Level 6: GenAI-Driven Innovation
At this stage, organizations rely on GenAI for ongoing product innovation, positioning AI as a critical source of competitive advantage.
New AI-powered products and services emerge, supported by strategic decision-making informed by GenAI analytics.
Companies actively contribute to the GenAI ecosystem, often through collaborations or open-source contributions, reinforcing a culture of AI-driven innovation.
Organizations can establish partnerships with research institutions, refine continuous improvement cycles, and expand AI-driven offerings into new markets or service areas to continue advancing.
Level 7: GenAI Leadership
At the highest maturity level, organizations lead in GenAI, shaping the technology’s future and its role in the market.
These companies set industry standards, contribute to GenAI research, influence policy, and advance responsible AI use.
As industry leaders, they actively engage in ethical debates and shape AI best practices, attracting top AI talent and fostering an innovation-centered culture.
To maintain their leadership, these companies often partner with academic institutions, participate in policy-making initiatives, and invest heavily in GenAI research, positioning themselves as pivotal players in the AI space.
Why On-Prem Solutions Are Essential for Advanced GenAI
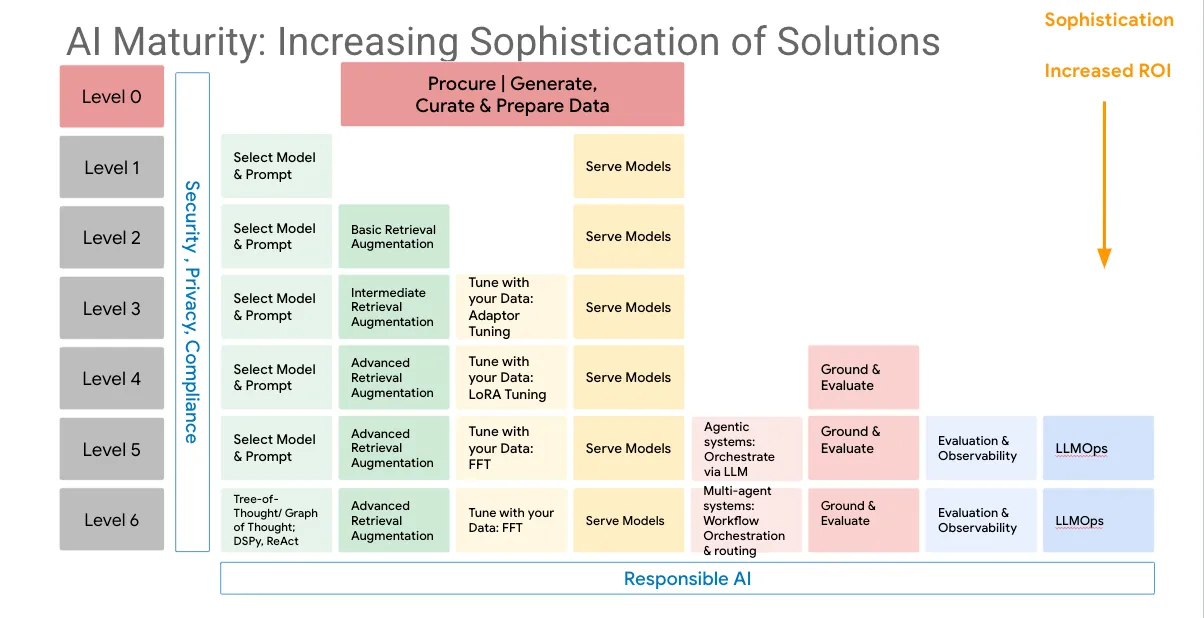
As organizations progress in their GenAI maturity, the infrastructure supporting these advanced capabilities must scale and adapt accordingly.
On-premises (on-prem) solutions are gaining traction, particularly among enterprises prioritizing control, security, and performance.
Here’s an in-depth look at how on-prem solutions uniquely support advanced GenAI deployments.
Security and Data Privacy
On-prem solutions provide unparalleled data security and privacy control, a critical advantage for companies dealing with sensitive information.
By keeping GenAI systems on internal servers, organizations mitigate risks associated with data breaches, third-party access, and cloud network vulnerabilities.
On-prem deployments are particularly beneficial in highly regulated industries like healthcare and finance, where frameworks such as GDPR (General Data Protection Regulation) and HIPAA (Health Insurance Portability and Accountability Act) impose stringent data handling and storage requirements.
With on-prem solutions, organizations retain full oversight of data flows, ensuring compliance with regulatory standards that demand strict data residency and sovereignty protocols.
Moreover, on-prem infrastructure can facilitate regular, custom security audits tailored to organizational needs. This flexibility supports rapid adjustments to evolving compliance standards without the latency of external cloud service policies.
As regulations surrounding AI and data privacy continue to evolve, on-prem solutions offer a secure and adaptable foundation for GenAI applications in sensitive environments.
Latency and Performance
Latency can be a critical concern for high-performance GenAI tasks.
In real-time applications like financial trading, telemedicine, or autonomous driving, the milliseconds required to transfer data to and from a cloud server can make a substantial difference.
On-prem deployments eliminate this delay by keeping processing operations local, directly boosting system responsiveness and operational speed.
Latency reduction is especially important as GenAI models grow in complexity, demanding intensive computing resources and faster processing.
For instance, microsecond delays in high-frequency trading can significantly impact decision-making, making on-prem solutions vital for organizations reliant on real-time data and analytics.
Furthermore, with on-prem infrastructure, companies can fully leverage advanced hardware such as GPUs (Graphics Processing Units) and TPUs (Tensor Processing Units) optimized specifically for AI workloads.
Direct control over this hardware enables organizations to customize configurations for optimal GenAI performance, maximizing computational power and minimizing processing bottlenecks.
Cost-Effectiveness for Scale
While cloud solutions offer flexibility and scalability, scaling GenAI on-prem can be more cost-effective in the long term, particularly for organizations with consistently high processing demands.
With cloud services, costs can escalate rapidly as the volume of data and processing power required grows, often exceeding initial budget expectations due to variable usage fees.
On-prem deployments, however, enable companies to make a one-time capital investment in infrastructure, which can be amortized over time.
This results in a lower total cost of ownership (TCO) for organizations requiring sustained, high-capacity GenAI processing.
This approach allows companies to avoid unpredictable costs associated with cloud scalability and instead budget for maintenance, upgrades, and expansion at a more predictable rate.
In addition, on-prem solutions reduce dependency on cloud providers, protecting organizations from future price hikes or service disruptions.
For enterprises with the resources to establish and manage their infrastructure, the long-term financial benefits of an on-prem approach often outweigh those of a pay-as-you-go cloud model, offering improved ROI and predictability in large-scale GenAI operations.
Industry-Specific Use Cases Across Maturity Levels
Generative AI maturity varies significantly across industries, with on-prem solutions allowing for precise control, enhanced security, and optimized resource management.
Below are examples of how financial services, healthcare, retail, and manufacturing leverage on-prem GenAI solutions at different maturity levels to meet their unique operational demands and compliance requirements.
Finance
In the financial sector, on-prem GenAI solutions are critical for high-stakes applications where data security and speed are paramount.
GenAI models are trained on sensitive transaction data for fraud detection to recognize unusual patterns or anomalies.
This involves high-frequency data analysis and rapid response capabilities, which benefit from the low-latency, secure environment of on-prem solutions.
Additionally, credit scoring systems leverage GenAI models trained on a range of financial data, from transaction histories to customer profiles, to produce dynamic, accurate credit assessments without exposing sensitive information to cloud vulnerabilities.
On-prem GenAI also strengthens compliance in handling customer data. Financial institutions are bound by regulatory requirements such as GDPR and PCI DSS, which necessitate strict data privacy and security controls.
By deploying GenAI solutions on-premises, these institutions ensure that sensitive data remains within their controlled infrastructure, reducing risks associated with data breaches and unauthorized access.
Healthcare
For healthcare providers, on-prem GenAI offers diagnostics, personalized medicine, and advantages in patient data management.
On-prem solutions enable healthcare organizations to maintain compliance with regulatory standards like HIPAA, safeguarding patient confidentiality and data integrity.
For instance, GenAI models used in diagnostic imaging analyze large volumes of sensitive medical images to identify conditions such as tumors, fractures, or other anomalies.
Storing and processing this data on-premises ensures its security, reducing the risks of transferring sensitive medical information to third-party servers.
GenAI can analyze genomic data in personalized medicine to recommend individualized treatment plans.
The sensitive nature of genetic information mandates the highest levels of security, which on-prem solutions effectively provide by keeping this data within the healthcare provider’s secure infrastructure.
On-prem solutions also support comprehensive patient data management by allowing healthcare providers to integrate and process patient information from various departments and sources, enabling a more holistic and secure approach to patient care.
Retail
On-premises GenAI solutions are instrumental in demand forecasting, pricing, and personalized marketing in retail.
For demand forecasting, GenAI models analyze historical sales data, seasonal trends, and regional preferences to accurately predict customer demand.
By deploying these models on-premises, retailers maintain full control over their proprietary sales data, a competitive industry asset.
Pricing strategies benefit from GenAI’s ability to assess competitor pricing, customer behavior, and market trends in real-time.
With on-prem solutions, retailers can adjust prices dynamically across locations and product lines without risking data exposure to cloud-based vulnerabilities.
In personalized marketing, on-prem GenAI enables retailers to analyze purchase history and customer preferences to tailor product recommendations and promotions.
Processing this customer data on-premises enhances data security and helps retailers comply with regulations like GDPR by keeping personal data secure and within jurisdictional boundaries.
Manufacturing
In manufacturing, on-prem GenAI supports applications like predictive maintenance, inventory management, and supply chain optimization.
For predictive maintenance, on-prem GenAI models process sensor data from machinery to anticipate failures and schedule timely repairs, reducing downtime and optimizing operational efficiency.
This real-time data processing requires low latency, which is best achieved with on-prem solutions, particularly in industrial environments where rapid data processing can directly impact productivity.
Inventory management benefits from GenAI’s ability to forecast demand, optimize stock levels, and prevent overproduction.
On-prem solutions allow manufacturers to securely store and analyze proprietary inventory data without relying on external cloud services.
Additionally, supply chain optimization leverages GenAI to track and analyze complex logistics data, such as shipping routes, supplier performance, and lead times.
Keeping this data on-premises ensures better control over sensitive supply chain information, critical in industries like automotive or electronics, where proprietary manufacturing processes are highly guarded.
Future Trends in GenAI Maturity and On-Prem Technologies
As Generative AI (GenAI) continues to mature, on-premises deployments are positioned to play an increasingly strategic role, especially as new capabilities and requirements emerge in AI technology.
Here, we explore three critical trends likely to shape the future of GenAI in on-prem environments:
Multi-Agent Systems and Edge AI
A notable trend in AI development is the shift toward multi-agent systems—deployments where multiple specialized AI models, or “agents,” work together to solve complex problems.
These systems enhance efficiency and decision-making accuracy by enabling various models to interact, share insights, and delegate tasks.
For on-prem GenAI, multi-agent systems offer substantial benefits by allowing organizations to compartmentalize tasks across localized models. This ensures that each AI component is focused on a particular function while securely housed within the organization’s infrastructure.
Edge AI, another emerging trend, is also transforming the landscape by moving AI processing closer to data sources, thereby reducing latency and improving response times.
On-prem GenAI can leverage edge AI to decentralize processing, enabling faster, real-time decision-making in settings like manufacturing and healthcare where immediate insights are essential.
Together, multi-agent systems and edge AI promise to increase the speed, accuracy, and security of on-prem GenAI implementations by optimizing performance locally and minimizing the need for data transfer to external, cloud-based locations.
Enhanced Data Pipelines and Infrastructure
The success of GenAI hinges on efficient data flow and processing, which means that future advancements in on-prem technology will focus heavily on data pipeline optimization and high-performance infrastructure.
As organizations increasingly deploy on-prem GenAI, they will likely invest in next-generation data pipelines capable of handling massive volumes of structured and unstructured data with minimal lag.
Innovations in pipeline architecture, such as intelligent data orchestration and parallel processing, can streamline data movement and transform how information flows between storage and processing units.
Furthermore, advances in high-performance computing (HPC) solutions tailored for GenAI will become more prevalent in on-prem environments.
These HPC advancements—characterized by faster GPUs, scalable storage options, and robust networking solutions—will make it easier for organizations to train and deploy large GenAI models without relying on external resources.
By continuously investing in efficient data pipelines and high-capacity infrastructure, on-prem solutions are poised to support GenAI workloads with unparalleled reliability and speed, offering a scalable foundation that aligns with growing AI demands.
Ethical and Responsible AI
As the regulatory landscape around AI solidifies, on-prem solutions may become central to ensuring compliance with ethical standards and responsible AI practices.
Emerging guidelines, such as those under development by global data protection authorities, will likely enforce stricter controls on AI model usage, data handling, and bias mitigation.
For organizations that must comply with specific ethical standards, on-prem solutions offer a secure, controlled environment where data governance and model integrity can be closely managed.
Additionally, on-prem solutions empower organizations to implement “explainable AI” practices, essential for meeting transparency standards.
Since on-prem deployments allow organizations to directly oversee their GenAI models, they can establish more robust monitoring, auditing, and documentation processes.
This capability positions on-prem GenAI as a reliable choice for industries where transparency and accountability are important, such as finance and healthcare.
On-prem solutions can help organizations align their AI strategies with future regulations and societal expectations by supporting ethical and responsible AI.
Transitioning to On-Prem Deployment: Strategic Considerations
Transitioning to an on-premise GenAI deployment is a complex but rewarding journey for organizations prioritizing control, compliance, and performance in their AI operations. Successful implementation demands careful evaluation of readiness, robust infrastructure, a skilled internal talent base, and continuous optimization.
Assessing Readiness for On-Prem GenAI
Determining an organization’s preparedness for on-prem deployment begins with evaluating several critical factors.
First, data scale and storage requirements are paramount; organizations must ensure they can support the extensive data volumes necessary for GenAI.
Regulatory needs are also a priority, particularly for industries like healthcare and finance, where data protection regulations such as GDPR and HIPAA mandate strict control over data handling.
Additionally, understanding an organization’s usage patterns and processing needs, including peak demand periods and real-time analytics requirements, helps establish whether on-prem deployment aligns with operational goals and enhances system resilience.
Infrastructure Requirements and Cybersecurity
A successful on-prem deployment relies heavily on a robust infrastructure designed to handle the unique demands of GenAI systems.
High-performance hardware, including advanced GPUs and storage systems like NAS or SAN, is essential to support the intense computational and storage needs of GenAI workloads. Network architecture must accommodate high data throughput and minimize latency, ensuring efficient and reliable operations.
Cybersecurity measures play a crucial role in safeguarding sensitive data within on-prem environments. Implementing comprehensive security protocols, including end-to-end encryption, firewalls, and regular security audits, establishes a secure foundation for GenAI.
Data management and governance practices must also be in place to ensure compliance with regulatory standards, optimize data handling, and support efficient retrieval and analysis.
Building an Internal GenAI Talent Base
The transition to an on-prem GenAI deployment necessitates a skilled workforce capable of managing, maintaining, and optimizing the system.
Developing a GenAI talent base involves upskilling current employees and recruiting specialized roles.
Skills development focuses on areas like data science, machine learning, data engineering, and cybersecurity, empowering existing team members to support GenAI operations effectively.
Recruiting AI infrastructure architects, cybersecurity specialists, and data governance experts further strengthens the organization’s ability to maintain a resilient GenAI environment.
Cross-functional training fosters collaboration between data scientists, engineers, and security experts, enabling a holistic system management and optimization approach.
Continuous Optimization and Evaluation
On-prem GenAI deployments require ongoing evaluation and fine-tuning to maintain optimal performance and adaptability.
Performance monitoring involves tracking key metrics such as latency and processing speed, enabling the organization to adjust as needed.
Regular security audits are essential to staying ahead of evolving cybersecurity threats, helping to identify and mitigate potential vulnerabilities.
Periodic model tuning is another critical component, allowing organizations to enhance model accuracy and efficiency by refining data pipelines and updating hardware resources based on real-time usage data.
Establishing feedback loops with end-users and implementing system updates ensures that the infrastructure remains responsive to changing requirements and continuously aligns with the organization’s business objectives and technological advancements.
Work Smart & Intelligently with Knapsack
Boost your productivity and streamline your workflows with Knapsack.
Knapsack empowers teams with automation and data-driven insights, allowing them to focus on strategic goals while reducing manual processes.
With customizable dashboards, seamless integrations, and intelligent data processing, Knapsack is the ideal solution for enhancing efficiency and achieving impactful results.