Will AI Replace Data Analysts?
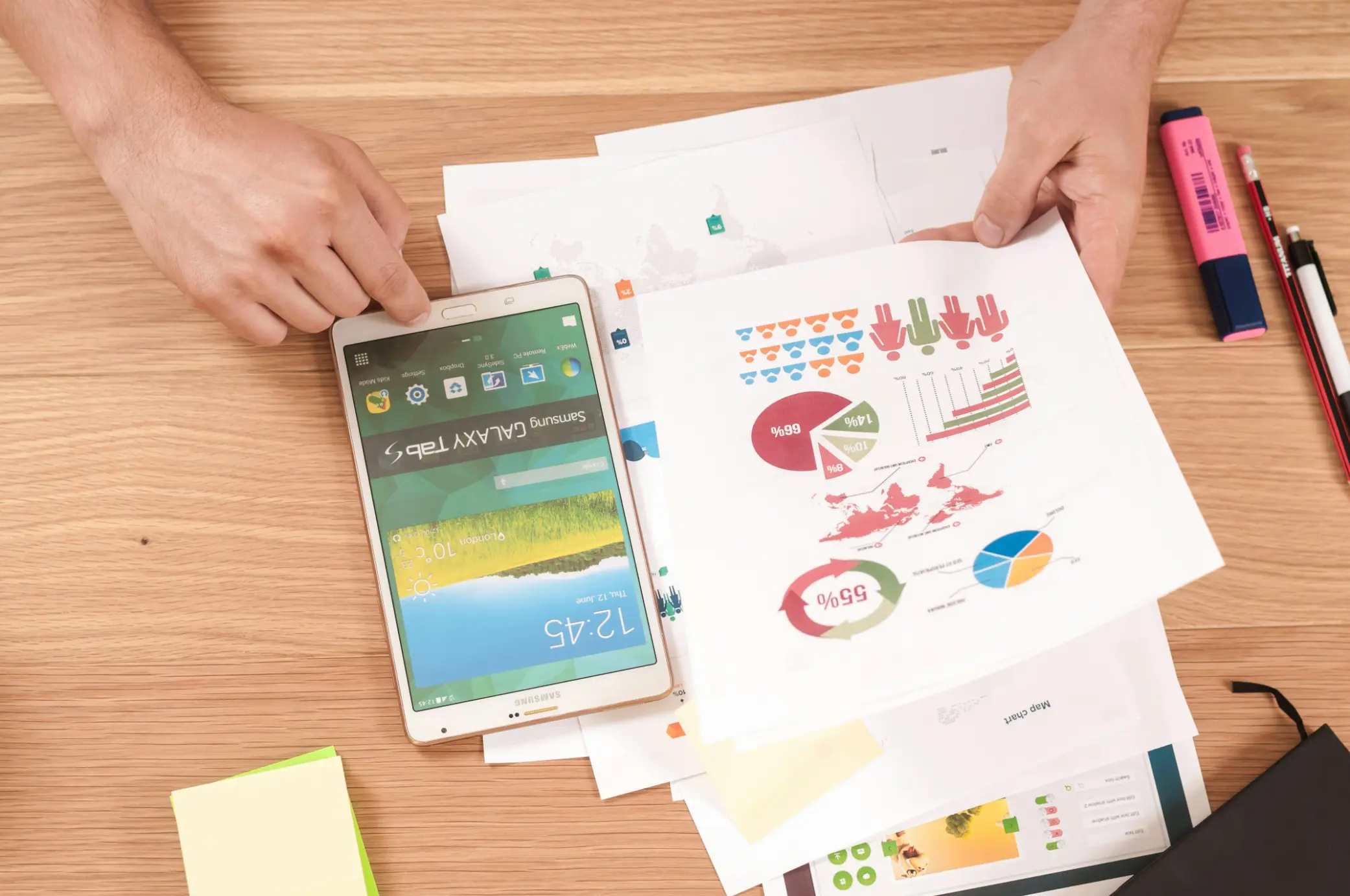
Artificial intelligence (AI) has significantly transformed various industries, and data analytics is no exception. With advancements in machine learning and automation, many data analysts are now questioning whether AI will soon replace their roles.
While AI is poised to automate many routine tasks, the role of human data analysts is far from obsolete. Instead of replacing them, AI is more likely to augment their capabilities, enabling them to focus on more strategic, value-added activities.
In this article, we will explore how AI is transforming the data analytics profession, how the role of data analysts is evolving, and what the future holds for professionals in this field.
How AI is Currently Used in Data Analysis
Artificial intelligence plays a crucial role in the data analytics landscape, automating repetitive tasks, improving data processing speed, and providing deeper insights. While AI can handle routine aspects of data analysis, human analysts remain essential for interpreting the results and applying business context to AI-generated outputs.
Automating Routine Tasks
One primary way AI is transforming data analysis is by automating routine and time-consuming tasks. AI-powered tools are now handling tasks like data cleaning, data preparation, and basic data visualization.
AI algorithms can sift through vast amounts of raw data, identify inconsistencies, clean the data, and prepare it for analysis much faster than humans. This automation frees data analysts from spending hours on mundane tasks, allowing them to focus on more complex and strategic work.
Gartner predicted that more than 40% of data science tasks would be automated by 2020, increasing industry productivity. This trend has empowered "citizen data scientists," enabling more employees to access and analyze data with minimal technical expertise.
Enhancing Data Analysis Capabilities
AI speeds up the process and enhances the overall capabilities of data analysis. Machine learning models can analyze vast amounts of structured and unstructured data at speeds beyond human capacity. These models can identify patterns and trends that may not be immediately apparent to human analysts, providing richer insights into the data.
AI is particularly valuable in predictive analytics, where models are trained on historical data to forecast future trends and outcomes.
For example, in finance, AI-driven predictive analytics is used to predict market movements and assess risks more accurately than traditional methods. As AI tools become more sophisticated, they will continue to augment analysts' capabilities by generating insights that lead to more informed decision-making.
Improving Decision-Making with AI
AI has revolutionized real-time decision-making by providing instant insights based on data analysis. AI tools can process data continuously and generate insights in real-time, allowing businesses to make quicker and more informed decisions. For instance, AI models can provide instant feedback on market conditions, helping financial firms adjust their strategies immediately.
The AI market is expected to grow by 37% in 2024, further increasing its adoption across various sectors, including data analytics. The increasing reliance on AI for real-time decision-making highlights its importance in supporting data-driven strategies across industries.
Limitations of AI Without Human Analysts
While AI is excellent at automating processes and analyzing data, it lacks the nuanced understanding of context that human analysts provide. AI can identify patterns and correlations, but human analysts must interpret these results, draw meaningful conclusions, and apply them to specific business contexts. For example, AI might highlight an anomaly in sales data. Still, a human analyst must determine whether it is due to a seasonal fluctuation, a marketing campaign, or an external economic factor.
Moreover, AI models are only as good as the data on which they are trained. Bias in the data or incomplete datasets can lead to misleading results, which human analysts need to detect and adjust. Therefore, while AI enhances the efficiency and accuracy of data analysis, it still requires human oversight to ensure that insights are relevant and actionable.
Evolving Role of Data Analysts in the AI Era
As artificial intelligence becomes more prevalent in data analytics, the role of data analysts is shifting. Instead of being replaced by AI, data analysts are evolving their responsibilities to incorporate new tools and strategies that enhance their capabilities. Rather than automating away their jobs, AI is transforming their work, allowing them to focus on higher-level tasks involving strategy and interpretation.
Augmenting, Not Replacing
While AI can handle many repetitive tasks that data analysts once performed, it is not expected to replace them fully. Instead, AI is seen as a tool that augments analysts' abilities, freeing them from mundane data preparation tasks and enabling them to focus on more complex analysis and decision-making.
According to recent surveys, 82% of analysts believe AI will enhance their roles, allowing them to spend more time on strategic work. This demonstrates that most professionals in the field view AI as an asset, not a threat to job security.
Demand for Data Analysts in the Job Market
Despite the rise of AI, demand for data analysts remains strong. The U.S. Bureau of Labor Statistics projects that the demand for data analysts will grow by 23% between 2021 and 2031. This growth is fueled by businesses’ increasing reliance on data-driven insights to inform their strategies. AI may automate some tasks, but human data analysts are still required to interpret results, provide business context, and ensure that the insights generated by AI are actionable.
Changing Responsibilities
As AI takes over more routine tasks, data analysts are called upon to take on higher-level responsibilities. These include interpreting AI-generated insights, communicating findings to stakeholders, and integrating AI tools into business workflows.
Analysts who can work with AI and understand its outputs will be in high demand, especially as businesses look for ways to maximize the value of their data. This shift is already visible, with many data analysts moving away from manual data processing toward roles that involve strategy, business intelligence, and AI model validation.
New Skills for the Future
Data analysts must develop new skills to work alongside AI tools to remain competitive in the evolving job market. These skills include proficiency in machine learning, natural language processing, and AI-driven analytics.
Analysts who can effectively use AI tools to enhance their analyses will be better positioned for the future. Additionally, understanding how to interpret and contextualize AI outputs is becoming critical. AI can often provide data without the business context, which is crucial for making informed decisions.
Benefits of AI in Data Analytics
The integration of artificial intelligence into data analytics brings numerous benefits that improve efficiency and enable more in-depth and actionable insights. By automating routine tasks and enhancing the analytical capabilities of data professionals, AI has enabled organizations to make faster, data-driven decisions.
Increased Efficiency and Speed
AI significantly increases the speed at which data can be processed and analyzed. Tasks that once took hours or even days for human analysts to complete, such as data cleaning, data wrangling, and basic visualizations, can now be performed in minutes using AI-driven tools. This allows data teams to work more efficiently and focus on delivering insights rather than spending time on repetitive tasks.
For instance, AI tools can automatically detect and clean missing or inconsistent data points, preparing datasets for further analysis in a fraction of the time it would take for a human analyst. This boosts the overall productivity of data teams, enabling them to handle larger and more complex datasets without sacrificing accuracy.
Enhanced Predictive Analytics
AI’s predictive capabilities are one of its most powerful contributions to data analytics. Machine learning models can analyze historical data and recognize patterns that may not be apparent to human analysts. These models can then use those patterns to forecast future trends and outcomes, giving businesses a competitive advantage.
For example, in industries like finance and marketing, AI-powered predictive analytics can forecast customer behavior, helping businesses plan more effective strategies. These models provide a higher accuracy level than traditional statistical methods, and they can continuously improve over time as more data is introduced.
Reducing Human Error
Another major advantage of AI is its ability to reduce human error in data processing and analysis. AI systems operate based on predefined rules and algorithms, ensuring consistent application of data models and reducing the risk of mistakes in manual processes. This is especially important when dealing with large datasets where data preparation or analysis errors could lead to inaccurate results.
By automating routine tasks and applying consistent data cleaning methods, AI minimizes the risk of errors and ensures that the insights derived from the data are accurate and reliable.
Democratization of Data Analysis
With AI tools becoming more accessible, data analysis is no longer confined to data specialists. AI-driven platforms have democratized data access by enabling non-technical users to perform basic data analyses without deep expertise in data science. These user-friendly AI tools allow employees across various departments to engage with data and generate insights, making data-driven decision-making a broader organizational practice.
This democratization empowers more people to participate in data analysis, spreading the benefits of data-driven insights throughout the organization. It also reduces the burden on data teams, as business units can handle more routine analysis tasks using AI tools.
Challenges of AI in Data Analysis
While artificial intelligence offers significant benefits to data analytics, it also presents several challenges. These challenges revolve around ethical concerns, reliance on AI without human oversight, the limits of AI’s understanding, and the need for continuous skill development in data teams.
Ethical Concerns and Bias
One of the most pressing challenges in using AI for data analysis is ensuring ethical use and managing bias in AI models. AI models are trained on historical data, and if this data contains biases, the AI can inadvertently replicate or amplify those biases in its outputs. This is particularly concerning in the finance, healthcare, and hiring sectors, where biased decisions can have serious consequences.
Data privacy and ethical considerations must be considered when using AI to process sensitive information. Organizations need to implement strict guidelines to ensure that AI is being used responsibly and that decisions made by AI systems are fair and unbiased.
Over-Reliance on AI
Another challenge is the risk of over-reliance on AI tools without sufficient human oversight. While AI can automate many tasks, it cannot fully replace the critical thinking and contextual understanding that human data analysts bring. Over-reliance on AI can lead to blind spots, especially in complex decision-making processes that require human judgment.
AI models can sometimes produce results that seem correct but lack the nuance needed to make strategic decisions. Data analysts must remain involved in verifying AI’s outputs and ensuring that the insights are aligned with business objectives and reality.
AI Lacks Contextual Understanding
Although AI excels at identifying patterns and correlations in large datasets, it lacks the broader contextual understanding that human analysts provide. AI can detect a trend or anomaly in the data but often struggles to explain why that trend occurs. For example, an AI model might identify a sudden spike in sales but would not necessarily understand that the spike is due to a marketing campaign or an external economic factor.
This lack of contextual understanding limits AI’s ability to make informed recommendations without human input. Human analysts must interpret the results, provide context, and apply the insights to real-world situations.
Skill Gaps in AI Adoption
As AI becomes more integrated into data analytics, data professionals must continuously update their skills to keep up with the latest technologies. This challenges organizations, as not all data analysts are currently equipped with the knowledge needed to work effectively alongside AI tools.
Data analysts must develop new skills in machine learning, natural language processing, and AI-driven analytics. Organizations must invest in ongoing training and development to ensure that their teams can effectively leverage AI tools and maintain their competitive edge.
Future Outlook for Data Analysts in an AI-Driven World
As AI continues to evolve and integrate into various industries, the future for data analysts looks promising yet requires adaptation. While AI will automate many tasks traditionally performed by data analysts, it is expected to augment rather than fully replace their roles. Analysts who combine their domain expertise with AI-driven insights will be well-positioned to thrive in the future.
AI and Human Collaboration
The future of data analysis lies in the collaboration between AI and human analysts. AI excels at processing large datasets quickly and identifying patterns that humans might miss.
However, human analysts will continue to be essential for interpreting these results, providing contextual insights, and guiding strategic decisions. AI will handle the heavy lifting, but humans will remain the key decision-makers.
This collaborative approach allows data analysts to move away from time-consuming tasks like data wrangling and instead focus on higher-level analysis. By leveraging AI tools, analysts can generate deeper insights, improve the accuracy of their predictions, and deliver more strategic recommendations. In this sense, AI empowers data analysts to be more effective and valuable to their organizations.
Continuous Learning for Analysts
As AI technologies evolve, the skill sets required for data analysts will also change. Analysts will need to engage in continuous learning to stay up-to-date with new tools and techniques. Developing expertise in machine learning, natural language processing, and real-time data analytics will become increasingly important.
Lifelong learning will be crucial for data professionals who want to remain competitive in the job market. Organizations are likely to prioritize hiring data analysts who are skilled in traditional data analysis and capable of working alongside AI-driven systems to derive insights from complex datasets.
Hybrid Roles of Analysts
The future will likely see the rise of hybrid roles where data analysts become "data strategists" or "data scientists," combining their traditional analytical skills with advanced AI and machine learning techniques. These hybrid roles will focus more on interpreting AI-generated insights, training AI models, and ensuring that AI systems are aligned with business objectives.
Data analysts will often evolve into AI specialists who can fine-tune models, apply them to specific business problems, and communicate AI-generated insights to non-technical stakeholders. This shift will make data analysts even more integral to organizations as they bridge the gap between technical AI tools and real-world business applications.
Boost Your Productivity with Knapsack
As AI continues to reshape the role of data analysts, professionals must stay ahead of the curve by leveraging tools that enhance productivity and streamline workflows. Knapsack is designed to support data analysts and AI teams by offering a robust platform that automates repetitive tasks, facilitates collaboration, and integrates AI tools to deliver deeper, actionable insights.
With Knapsack, data analysts can focus on more strategic analysis, spending less time on manual data preparation and more time deriving business-critical insights. Knapsack ensures that your data analysis process is efficient, scalable, and aligned with the latest AI advancements.
Boost your productivity with Knapsack and take your data analytics capabilities to the next level by simplifying your workflows and improving decision-making efficiency.