Benefits of Using AI for Data Analytics
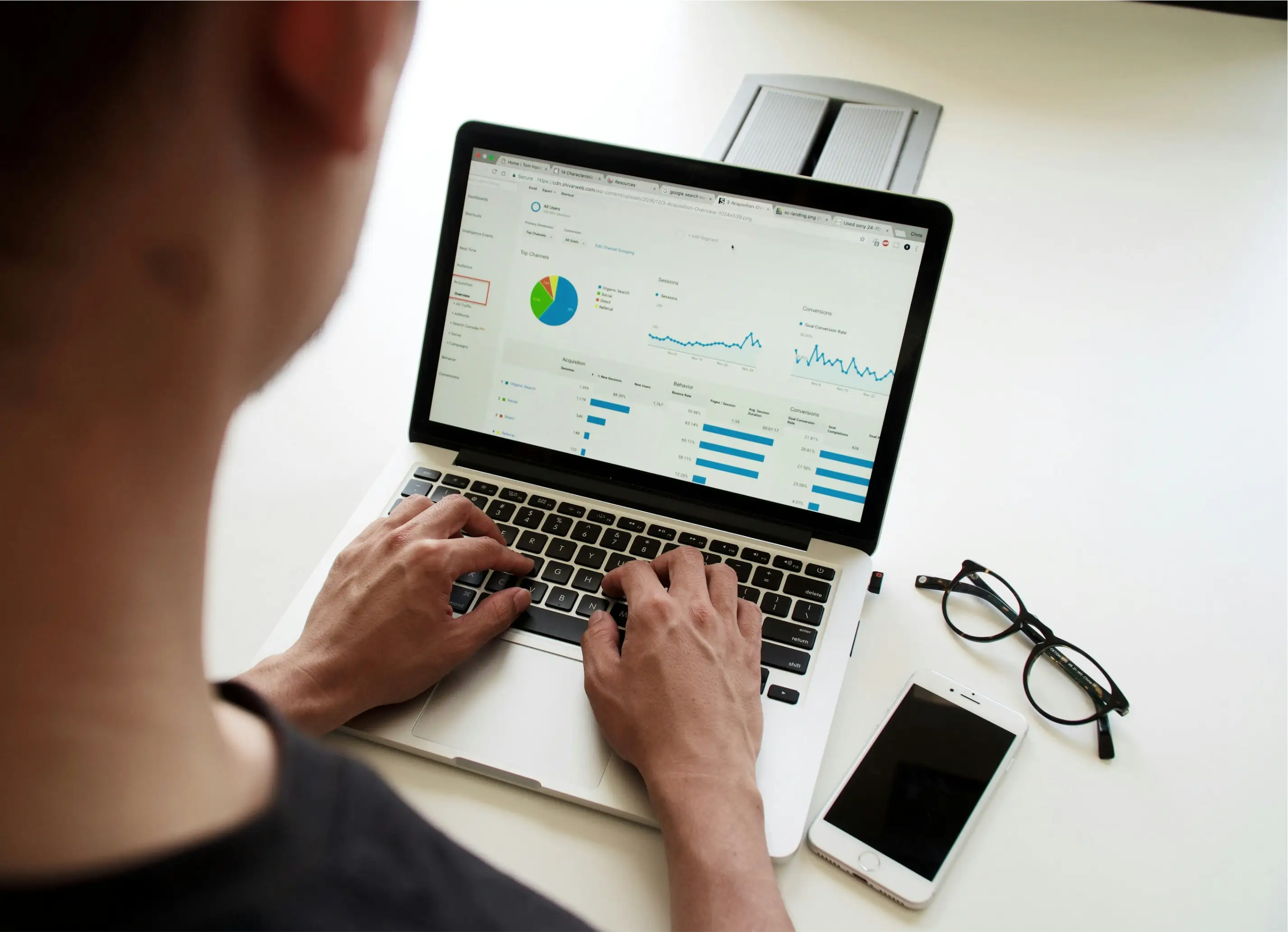
The power of artificial intelligence (AI) is transforming data analytics, making it faster, more accurate, and accessible across industries.
From small startups to large corporations, businesses are leveraging AI to extract actionable insights from vast datasets and make smarter, data-driven decisions.
This article will explore the key benefits of using AI in data analytics, focusing on how AI enhances speed, accuracy, and predictive capabilities while also reducing costs and effortlessly scaling with data needs.
Incorporating AI into data analytics offers companies a competitive advantage and a fundamental shift in how they operate, respond to market demands, and grow.
How Does AI Improve Efficiency in Data Analytics?
AI-driven tools are fundamentally transforming efficiency in data analytics by automating repetitive tasks, accelerating data processing, and reducing the manual workload for analysts.
These advancements allow organizations to derive insights faster, make timely decisions, and stay agile in a data-driven world.
Here are specific ways AI enhances efficiency in data analytics.
Rapid Data Processing for Timely Insights
AI algorithms can analyze large datasets far quicker than traditional methods, transforming hours or even days of manual analysis into minutes.
With AI, organizations gain near-instantaneous insights, enabling them to respond immediately to market changes, customer needs, or operational shifts.
For instance, in industries like finance and retail, where decisions need to be timely, AI’s rapid processing abilities give companies a significant advantage.
This speed allows organizations to react faster and explore “what-if” scenarios in real-time, testing potential strategies before implementing them.
Automation of Repetitive Tasks Like Data Cleaning and Preparation
Data preparation can consume significant time and resources, including cleaning, sorting, and categorizing.
AI automates these repetitive tasks by detecting inconsistencies, filling gaps, and categorizing data based on defined parameters.
This automation allows data professionals to focus on strategic analysis rather than manual data handling, increasing productivity and job satisfaction.
A McKinsey study found that companies using AI for data preparation experienced a 30% improvement in efficiency, highlighting how automation reduces the labor-intensive aspects of data management.
Real-Time Analytics for Fast-Paced Decision-Making
AI’s capability to provide real-time analytics is invaluable for organizations operating in dynamic environments.
Real-time insights enable companies to monitor key metrics as they evolve, allowing decision-makers to react swiftly to emerging trends or operational changes.
For instance, real-time analytics in e-commerce allows businesses to adjust marketing efforts based on current customer behavior, while in manufacturing, real-time data from AI systems can signal equipment malfunctions before they impact production.
This ability to act on current data improves agility and keeps companies aligned with immediate demands.
AI’s Role in Streamlining Workflows and Reducing Bottlenecks
AI optimizes workflows by automating parts of the data pipeline, ensuring that each phase flows without unnecessary delays, from data collection to analysis.
For instance, AI tools can automate data integration from multiple sources, making the process seamless and reducing data transfer bottlenecks.
By streamlining workflows, AI helps organizations maintain momentum, especially in high-volume data environments.
This efficiency allows teams to handle larger datasets without a proportional increase in time or labor, directly impacting operational productivity.
A well-known example of AI-driven efficiency is in the finance sector, where AI systems analyze transaction data in real-time to detect fraud.
AI algorithms monitor thousands of transactions per second, identifying suspicious activities that could signal fraud enabling instant responses that protect assets.
In retail, AI-driven analytics allows companies to instantly personalize customer experiences by analyzing purchasing behavior, leading to more effective and targeted marketing campaigns.
What Are the Accuracy and Quality Benefits of AI in Data Analytics?
Accuracy and data quality are foundational to effective analytics, and AI has emerged as a powerful tool for improving both.
By automating error detection, validating data consistency, and minimizing human biases, AI ensures that data analytics outcomes are accurate and reliable.
Here’s how AI enhances data accuracy and quality, leading to more informed decision-making.
Automated Data Validation for Consistency
AI automates data validation by scanning datasets for inconsistencies, missing values, and duplicates that could otherwise compromise analysis.
AI identifies and corrects anomalies through machine learning algorithms, ensuring data is clean and standardized before it’s used for analysis.
This level of automation minimizes human intervention, which reduces errors typically associated with manual data checks.
For example, in healthcare, where data integrity is crucial, AI-driven validation has improved patient data accuracy by up to 30%, leading to more reliable diagnostic insights and treatment plans.
Reduction of Human Error in Analytics
Human errors in data handling, such as input mistakes or incorrect labeling, can distort analytics results.
AI minimizes these risks by automating routine tasks and establishing strict data processing protocols that adhere to predefined standards.
Since AI operates without the fatigue or oversight issues that may affect human analysts, it ensures consistency across datasets.
Organizations that adopt AI for data handling report a 60% reduction in analysis errors, which improves data accuracy and bolsters trust in analytics-based decision-making.
Enhanced Data Reliability and Quality
AI enhances data quality by continuously learning and adapting from previous datasets, making it more effective at handling complex data environments over time.
Through this iterative learning process, AI refines its ability to identify and rectify data discrepancies, resulting in high-quality datasets that improve the accuracy of predictive models and analytical insights.
Companies leveraging AI in data validation processes have reported significant improvements in data quality, allowing them to make better-informed decisions backed by reliable analytics.
In finance, JP Morgan Chase implemented AI-driven data validation for transaction analysis to ensure accuracy in financial reporting.
By automating data checks and validations, the bank reduced reporting errors and achieved compliance with strict financial regulations.
This shift toward AI-enhanced accuracy saved time and improved the quality of insights delivered to decision-makers, showcasing the value of AI in elevating data reporting standards.
Mitigating Human Bias in Data Interpretation
Human biases in data interpretation can lead to skewed results that don’t accurately reflect underlying trends.
AI mitigates these biases by analyzing data through objective algorithms, which base conclusions on statistical patterns rather than subjective interpretation.
This capability is particularly valuable in fields like finance and hiring, where unbiased data analysis supports fairer and more accurate decision-making.
For example, AI algorithms used in recruitment have been shown to improve candidate selection accuracy by 40%, focusing purely on skill and experience metrics and avoiding human prejudices.
How Does AI Enable Advanced Predictive Analytics?
Predictive analytics is one of AI’s most powerful applications. It allows organizations to anticipate trends, forecast demand, and make proactive decisions based on data-driven predictions.
AI-driven predictive analytics uses sophisticated algorithms to identify patterns in historical data, generating insights that help companies stay ahead in fast-evolving markets.
Here are the key ways AI enhances predictive analytics and enables organizations to act on future-focused insights.
Predictive Modeling to Identify Future Trends
AI’s ability to recognize patterns in vast datasets allows it to create predictive models that accurately forecast future events.
For example, AI algorithms can analyze customer purchasing behavior to predict upcoming trends, enabling companies to adjust inventory or launch targeted marketing campaigns.
In industries like retail, predictive models allow businesses to align with consumer preferences proactively, while in finance, they help forecast market shifts, empowering organizations to make strategic investments.
A Deloitte study found that companies using AI for predictive modeling experienced a 25% improvement in forecast accuracy.
Real-Time Predictive Insights for Dynamic Environments
AI provides real-time predictive insights by continuously learning from new data as it’s collected, which is invaluable in fast-paced sectors where conditions can change rapidly.
This real-time capability enables businesses to adapt their strategies immediately, responding to trends or events as they happen.
For instance, real-time predictive analytics in logistics allows companies to optimize delivery routes based on live traffic data, minimizing delays and improving customer satisfaction.
This ability to react dynamically to present conditions gives organizations a competitive edge in industries requiring rapid adaptability.
AI-driven predictive maintenance has proven essential in minimizing equipment downtime and reducing maintenance costs in manufacturing.
By analyzing data from machinery sensors, AI can detect early signs of wear or potential failures, allowing companies to schedule maintenance before breakdowns occur.
This proactive approach prevents costly disruptions and extends equipment life, contributing to significant cost savings.
Companies implementing AI for predictive maintenance report up to 30% reductions in unplanned downtime, underscoring AI's impact on industrial efficiency.
Predictive Capabilities in Customer Behavior Forecasting
AI-powered predictive analytics can help companies understand and anticipate customer behavior, enabling them to tailor their marketing strategies more effectively.
By analyzing past interactions, AI can forecast customer preferences, predict purchase likelihood, and recommend personalized experiences that increase customer engagement and loyalty.
Retailers, for instance, use AI to predict which products are likely to appeal to certain demographics, creating more targeted promotions that drive sales.
A report from McKinsey noted that companies using predictive analytics for customer behavior saw a 20% increase in customer retention, illustrating the value of AI in maintaining strong customer relationships.
The Role of AI in Enhancing Resource Allocation
AI-driven predictive insights also aid in resource allocation by anticipating demand fluctuations, allowing organizations to optimize resources efficiently.
For example, AI can predict patient admission rates in healthcare, helping hospitals allocate staff and equipment effectively based on projected needs.
This optimized allocation prevents resource shortages and ensures organizations are prepared to meet demand without overextending budgets.
Companies can balance operational costs with service readiness through predictive analytics, maximizing productivity and reducing waste.
How Does AI Contribute to Cost Reduction in Data Analytics?
AI has proven invaluable in reducing costs associated with data analytics by automating labor-intensive tasks, optimizing resource usage, and minimizing operational expenses.
With AI-driven efficiencies, organizations can allocate resources more effectively and achieve substantial savings across various aspects of data management.
Here’s how AI specifically contributes to cost reduction in data analytics.
Reducing Labor Costs Through Automation
One of AI's most immediate cost-saving benefits in data analytics is its ability to automate repetitive and time-consuming tasks, such as data cleaning, sorting, and categorizing.
By handling these tasks without human intervention, AI reduces the need for large data teams to perform manual data processing.
For example, AI algorithms can process and validate data at speeds that would require significantly more human labor, freeing up analysts to focus on higher-value work.
A report from Accenture highlights that companies using AI-driven automation in data analytics can save up to 50% in labor costs, demonstrating AI’s potential to cut costs while enhancing productivity.
Optimizing Data Storage and Resource Usage
AI helps organizations optimize storage by categorizing and archiving data efficiently, ensuring that only essential data occupies high-cost storage resources.
AI-driven data management platforms can analyze usage patterns to determine which data should be archived or moved to cost-effective storage, minimizing unnecessary expenses.
In addition, AI’s ability to streamline resource allocation reduces the infrastructure costs associated with scaling analytics as data volumes grow.
This optimization decreases operational costs and allows companies to invest in data storage solutions that better suit their budgets and needs.
AI’s Role in Minimizing Data Migration Costs
Data migration can be expensive and complex, especially when consolidating large datasets or integrating new systems.
AI can reduce the need for data migration by enabling virtual data access, which allows users to query and analyze data from multiple sources without physically moving it.
This approach, known as data virtualization, helps organizations avoid the infrastructure and labor costs typically associated with data migration.
Studies show that companies using data virtualization tools report up to 70% savings in data access costs, as they eliminate the need for costly and time-intensive migrations.
Unilever, a global consumer goods company, adopted AI to enhance efficiency in its supply chain management. It uses predictive analytics to optimize inventory levels and reduce wastage.
By anticipating demand patterns and adjusting stock accordingly, Unilever minimized the costs associated with overstocking and underutilizing resources.
This AI-driven approach led to an estimated $100 million in annual savings for Unilever, underscoring AI's substantial cost-reduction potential when integrated into data-driven supply chain strategies.
Energy-Efficient Data Management with AI
AI-powered data management solutions also contribute to cost savings by optimizing energy usage, particularly in data centers.
AI systems monitor and adjust energy consumption by powering down idle servers, managing cooling systems, and shifting data to lower-energy storage solutions when possible.
These energy-efficient practices reduce electricity expenses, a significant part of operational costs for data centers.
By enhancing energy management, AI cuts costs and supports sustainability efforts, allowing companies to reduce their carbon footprint while achieving budgetary savings.
How Does AI Improve Scalability and Flexibility in Data Analytics?
AI is essential in helping organizations scale their data analytics efforts without proportionally increasing costs or labor.
With AI-driven solutions, companies can more effectively handle growing data volumes and adapt to new data sources, ensuring that their analytics remain efficient and responsive to business needs.
Here are specific ways AI enhances scalability and flexibility in data analytics.
Scalability for Handling Large Datasets Without Added Resources
One of AI’s core strengths in data analytics is its ability to process vast datasets efficiently, enabling organizations to analyze more data without requiring additional resources.
Machine learning algorithms can handle massive data inputs, analyzing them rapidly and delivering insights that would otherwise require significant computing power and human labor.
This scalability ensures that companies can expand their data operations in response to business growth or increasing data demands without scaling up their data teams.
For example, companies using AI for big data analytics report an 80% improvement in processing capacity compared to traditional methods, highlighting AI’s ability to manage larger data workloads effectively.
Adapting to Diverse Data Sources with Ease
AI enables organizations to seamlessly integrate and analyze data from multiple sources, allowing for a more comprehensive view of business metrics.
Whether data is structured, unstructured, or sourced from various departments, AI algorithms can process and standardize it for consistent analysis.
This flexibility is crucial in environments where data sources are constantly evolving, such as customer insights from social media, IoT device data, or real-time metrics from internal systems.
Organizations using AI to manage diverse data sources report smoother integration processes, as AI handles standardization and consolidation, minimizing the need for extensive manual data preparation.
AI’s Role in Integrating New Data Types Seamlessly
As businesses adopt new technologies and data types, such as video analytics, text from customer service logs, or geolocation data, AI is critical in incorporating these diverse data forms into analytics systems.
AI algorithms are designed to adapt to unstructured data, making it possible to analyze information that traditional analytics tools may overlook.
For instance, Natural Language Processing (NLP), a branch of AI, allows companies to analyze customer feedback, reviews, and social media posts to gain insights into customer sentiment and preferences.
By seamlessly integrating new data types, AI enhances the richness and relevance of analytics, allowing organizations to make data-driven decisions based on a more holistic view.
Enhanced Scalability in Fast-Growing Industries
In industries experiencing rapid growth, such as e-commerce, healthcare, and finance, AI’s scalability allows companies to keep pace with increasing data needs without overhauling their analytics infrastructure.
For example, e-commerce platforms rely on AI to manage spikes in customer data during peak shopping periods, ensuring that analytics systems operate smoothly under high demand.
In healthcare, AI efficiently processes large volumes of patient data for diagnostics and treatment recommendations, allowing facilities to expand services without overwhelming their systems.
This scalability empowers businesses to maintain consistent analytics quality and operational performance even as they scale.
Amazon, a leader in e-commerce, relies on AI-driven scalability to manage its extensive data ecosystem, which includes customer purchase data, browsing behavior, and shipping logistics.
By employing AI, Amazon efficiently processes this vast data volume, enabling personalized recommendations, inventory management, and dynamic pricing strategies.
Amazon’s success demonstrates how AI-driven scalability supports operational agility. It allows companies to handle high data volumes easily while maintaining a high level of customer experience.
Frequently Asked Questions
Here are frequently asked questions about using AI for data analytics:
How does AI help with data analysis?
AI automates repetitive tasks, identifies patterns in large datasets, and provides real-time insights for quicker and more accurate analysis. This allows organizations to base decisions on reliable, up-to-date information, and teams can focus on strategic initiatives instead of routine data processing.
What are 3 major benefits of using AI in software testing?
AI in software testing reduces manual efforts through automation, detects errors with high accuracy, and speeds up the testing process. These benefits help organizations launch products faster while ensuring reliability. AI-driven testing minimizes time-to-market and enhances software quality.
What are 3 benefits of AI?
AI increases efficiency by automating routine tasks, improves accuracy by reducing human error, and enhances decision-making through predictive insights. These advantages help organizations optimize operations and anticipate customer needs. AI’s predictive power supports proactive and data-driven strategies.
What are the benefits of AI in data management?
AI improves data management by ensuring data accuracy, optimizing storage costs, and automating error detection. This leads to more reliable, accessible, and cost-effective data handling. AI-driven management systems make data easy to access and maintain.
Boost Your Productivity With Knapsack
Ready to take your data analytics to the next level?
Knapsack offers powerful AI-driven solutions to streamline data management, automate routine tasks, and deliver actionable insights that fuel smarter decisions.
Discover how Knapsack can transform your workflow and enhance productivity by visiting the Knapsack website.