How Does AI Detection Work? Uncover the Process
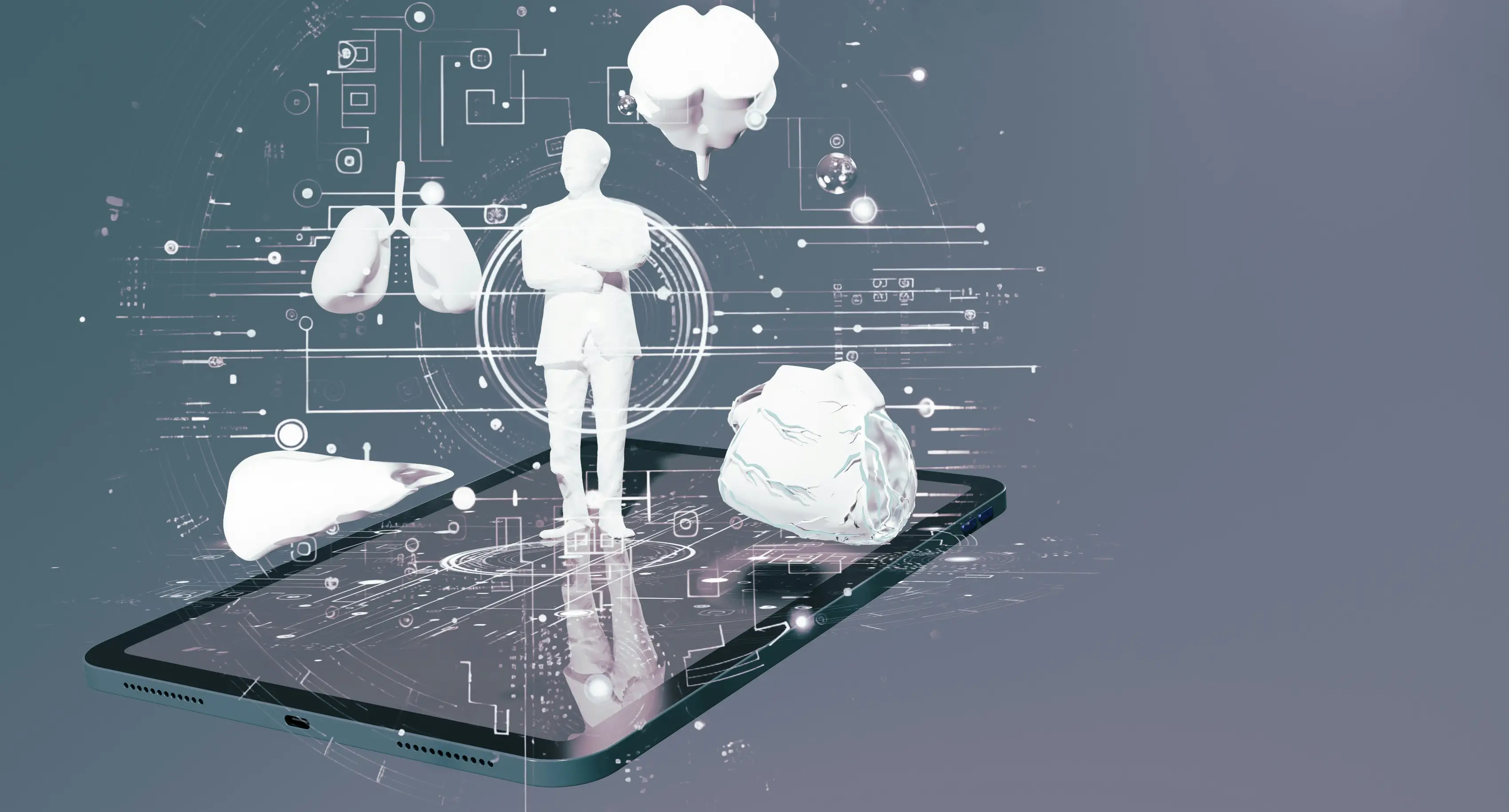
Detecting AI-generated content is becoming increasingly important as technology becomes a bigger part of our lives.
Knowing how AI detection works helps organizations stay secure and compliant in education, finance, or healthcare.
AI detection tools are designed to differentiate between human writing and AI-generated content.
This process ensures originality, prevents misinformation, and protects sensitive data.
In this article, you’ll learn what AI detection is, how it works, and why it matters for your industry.
What is AI Detection?
AI detection refers to tools and techniques designed to identify whether the content was created by artificial intelligence or a human author.
These tools have become essential as AI-generated content grows more sophisticated.
It is becoming harder to distinguish between machine-written and human-created text.
Understanding AI detection is vital in industries where originality, authenticity, and compliance are critical.
How AI Detection Works in Everyday Contexts
AI detection tools analyze text for patterns that indicate machine generation.
This includes examining grammar, syntax, and linguistic structures that differ from human writing.
For example, AI-generated content may lack idiomatic expressions or natural transitions.
These tools use complex algorithms to determine whether the writing aligns more closely with a human or AI style.
Why Is AI Detection Important?
The ability to detect AI content is crucial for maintaining data integrity and preventing misinformation.
In education, it ensures academic honesty by identifying AI-generated essays.
In finance and healthcare, detection tools help safeguard sensitive data and comply with strict regulations.
These tools protect businesses and individuals from the risks associated with unverified or artificially generated content.
Applications of AI Detection in Key Industries
AI detection is widely used across industries.
In education, tools like Turnitin ensure students submit original work.
AI detection helps identify fraudulent documents and prevent unauthorized content use in finance.
In healthcare, it verifies the authenticity of medical records and ensures compliance with data privacy standards like HIPAA.
The Growing Need for AI Detection
As AI models improve, they produce content that mimics human writing more closely.
This evolution has made detection tools indispensable in identifying machine-generated text.
The demand for accurate, reliable AI detection systems continues to grow across professional and academic sectors.
How Does AI Detection Identify AI-Generated Content?
AI detection tools use advanced techniques to pinpoint whether content was created by artificial intelligence.
These methods rely on analyzing patterns and characteristics typical of AI-generated text.
These tools help differentiate between human-written and machine-generated content by identifying linguistic markers, statistical features, and inconsistencies.
Linguistic Analysis Techniques and Their Challenges
AI detection tools examine the text for linguistic markers.
These include sentence structure, grammar, and style patterns.
AI-generated text often lacks human nuances, such as idiomatic expressions or cultural references.
Repetition of certain phrases or unusual combinations of words can indicate AI authorship.
However, as AI models improve, they mimic human writing more closely, making this method less reliable.
Statistical Approaches: Perplexity and Burstiness
Statistical metrics like perplexity and burstiness play a significant role in AI detection.
Perplexity measures how predictable a text is based on language patterns.
AI-generated text typically has lower perplexity because it is more formulaic and predictable.
Burstiness analyzes the variability in sentence length and structure.
Human writing tends to vary more, while AI-generated content often exhibits uniformity.
These metrics provide valuable clues but require updates as AI systems evolve.
Machine Learning Classifiers for Pattern Recognition
Machine learning classifiers are trained on large datasets of human-written and AI-generated text.
They analyze word choice, syntax, and tone to recognize patterns specific to AI writing.
These classifiers improve detection accuracy by identifying subtle differences in style and structure.
Regular updates to these systems are essential to keep pace with advancements in AI text generation.
Word Embeddings and Their Role in Content Analysis
Word embeddings represent words as vectors in a multidimensional space, capturing their meanings and relationships.
This method allows detection tools to analyze context and language subtleties.
AI-generated text often lacks the nuanced use of vocabulary seen in human writing.
For example, an AI might reuse specific phrases consistently, while a human writer tends to vary word choices.
Manual Reviews to Complement Detection Tools
Despite technological advancements, AI detection tools are not perfect.
Manual review by humans remains critical to validate flagged content.
Human oversight ensures context and intent are correctly interpreted.
Combining machine analysis and human expertise helps reduce false positives and negatives.
How Do AI Detection Tools Differentiate Human vs. AI Text?
AI detection tools use a combination of machine learning and linguistic analysis to distinguish between human and AI-generated content.
These tools recognize subtle patterns and stylistic elements that differentiate human writing from machine-generated text.
Training Machine Learning Classifiers with Datasets
Machine learning classifiers rely on extensive datasets containing human-written and AI-generated text examples.
These datasets help classifiers learn the differences in writing styles, vocabulary usage, and syntax.
For instance, human writing often reflects creativity and unpredictability, while AI-generated content follows more predictable patterns.
Training classifiers with diverse and up-to-date datasets ensures they remain effective as AI models evolve.
Role of Syntax, Grammar, and Semantic Relationships
AI detection tools analyze syntax, grammar, and semantics to identify discrepancies.
Human writing often exhibits more varied sentence structures and natural transitions between ideas.
AI-generated text, on the other hand, may have rigid sentence construction or awkward phrasing.
Detection systems use these linguistic cues to determine the likely origin of a text.
Challenges with Human-Edited AI Content
Human-edited AI content presents unique challenges for detection tools.
Humans modify AI-generated text by adding natural elements like idiomatic expressions or stylistic nuances.
These edits can mask the AI origin, making detection more difficult.
Tools must continually adapt to recognize hybrid content and accurately assess its authenticity.
Adapting Detection Tools to Evolving AI Models
AI models are constantly improving, producing text that closely mimics human writing.
Detection tools need regular updates to account for these advancements.
Without these updates, tools risk becoming ineffective as AI systems adopt more sophisticated techniques.
Staying ahead of AI developments is critical for reliable detection.
Types of AI Detection Techniques
AI detection employs various techniques to differentiate AI-generated content from human-written text. These methods combine linguistic analysis, statistical metrics, and machine learning algorithms to provide a comprehensive approach to content verification.
Analyzing Linguistic Patterns for Detection
Linguistic patterns are a key focus for AI detection tools.
These tools examine the text's coherence and structural elements, such as sentence flow and grammatical accuracy.
Repetitive phrases, unnatural word combinations, and rigid syntax are common indicators of AI authorship.
Pattern recognition techniques highlight these anomalies, helping to identify AI-generated content effectively.
Employing NLP for Syntax and Grammar Analysis
Natural Language Processing (NLP) tools are central in analyzing syntax and grammar.
NLP algorithms detect irregular sentence construction, awkward phrasing, or unnatural language transitions.
AI-generated text often exhibits linear and mechanical structures, while human writing tends to be more nuanced and expressive.
These tools enable detection systems to differentiate content based on linguistic sophistication.
Leveraging Perplexity and Burstiness Metrics
Perplexity and burstiness are statistical metrics used to assess text predictability and variation.
Low perplexity indicates that a text follows predictable patterns, often associated with AI generation.
Burstiness measures sentence length and complexity variability.
Human writing typically shows a high degree of burstiness, with varied sentence structures, while AI text tends to be more uniform.
These metrics help refine detection accuracy.
Cross-referencing with Existing Content Databases
AI detection tools often compare content against large databases of published material.
Tools can flag potential AI-generated content by identifying overlaps or similarities with existing texts.
For instance, if text replicates content from training data, it may be flagged as AI-authored.
This method, while effective, can lead to false positives when human writing coincidentally matches database material.
AI Detection in Multimedia: Images and Videos
Detection methods are not limited to text; they also apply to multimedia content like images and videos.
For example, AI-generated images are analyzed for pixel anomalies or watermarks embedded by creation tools.
Video detection examines frame sequences and audio-visual mismatches to identify synthetic content.
These methods expand AI detection's scope beyond text, addressing the broader challenge of identifying machine-generated media.
Challenges in AI Detection
AI detection systems face several challenges that limit their effectiveness and reliability. These challenges stem from the rapid evolution of AI models and the complexity of distinguishing human-authored content from machine-generated text.
Accuracy Limitations and the Problem of False Positives/Negatives
One of the primary challenges is accuracy.
Detection tools often misclassify content, flagging human-written text as AI-generated (false positives) or failing to detect AI-generated content (false negatives).
For example, repetitive or formulaic human writing, such as legal or technical documents, may be mistaken for AI content.
Conversely, advanced AI models can produce highly human-like text that goes undetected.
These inaccuracies undermine trust in detection systems and highlight the need for ongoing refinement.
Detecting Hybrid and Evasive AI-Generated Texts
Hybrid content, where human edits are applied to AI-generated text, is particularly challenging to detect.
Human modifications add stylistic nuances and variability, masking the AI origin.
Some AI systems are also designed to evade detection by mimicking human writing patterns more closely.
Detection tools must continuously adapt to identify these sophisticated evasive tactics.
Ethical Considerations and Balancing User Privacy
Ethical concerns arise when implementing AI detection tools, particularly in sensitive industries like healthcare and finance.
Ensuring that detection systems do not compromise user privacy or misuse data is crucial.
For instance, analyzing confidential documents may expose sensitive information, raising concerns about compliance with data protection regulations like HIPAA or GDPR.
Balancing the need for detection with ethical obligations is a critical challenge for organizations.
Lack of Standardized Frameworks for Detection
The absence of universal standards for defining and classifying AI-generated content complicates detection.
Different detection tools may use varying methodologies, leading to inconsistencies in results.
Standardized frameworks are essential to ensure reliable and comparable outcomes across industries and applications.
Overcoming Adversarial Attacks Against Detection Tools
Adversarial attacks, where content is deliberately manipulated to deceive detection systems, pose significant challenges.
For instance, subtle changes to text structure or word choice can confuse algorithms, resulting in misclassification.
Developers must implement robust defenses to counteract these tactics and ensure detection systems remain reliable.
Applications of AI Detection Across Industries
AI detection technologies are essential tools for enhancing security, ensuring authenticity, and maintaining compliance across various industries. These tools help organizations identify AI-generated content, protect sensitive information, and uphold ethical standards.
Use in Education to Ensure Academic Integrity
AI detection is widely used in educational settings to prevent academic dishonesty.
Tools like Turnitin analyze student submissions to identify AI-generated essays or assignments.
These tools ensure that students submit original work by detecting inconsistencies in writing style and structure.
This fosters a culture of honesty and accountability in academic institutions.
Role in Finance: Fraud Detection and Compliance
In the financial sector, AI detection helps identify fraudulent documents and communications.
These tools can analyze transaction records and contracts for anomalies, such as inconsistent language or unusual formatting.
This is critical for detecting manipulation or deceit in financial dealings.
AI detection also ensures compliance with regulations by verifying the authenticity of documents and communications.
Ensuring Authenticity in Healthcare Data
Healthcare relies on accurate and trustworthy documentation to deliver effective patient care.
AI detection tools scrutinize medical records, prescriptions, and clinical notes to ensure they are genuine and unaltered.
This is essential for maintaining patient safety and adhering to data privacy standards like HIPAA.
Detection tools reinforce trust between providers and patients by verifying the authenticity of healthcare data.
Enhancing Security Against Phishing and Cyber Threats
AI detection plays a crucial role in identifying phishing attempts and cyberattacks.
For example, detection tools analyze emails and communications for patterns indicative of AI generation, such as overly formal language or repetitive structures.
Promoting Ethical AI Use in Sensitive Industries
AI detection ensures the ethical use of technology in sectors like finance and healthcare.
Organizations can monitor and control the application of AI to prevent unauthorized or unethical usage.
This aligns with industry standards and fosters transparency and accountability in AI-assisted processes.
How to Integrate AI Detection into Professional Workflows
Integrating AI detection tools into professional workflows enhances data security, ensures compliance, and promotes ethical technology use.
Successful implementation requires careful planning, regular updates, and a combination of automated tools and human oversight.
Selecting the Right AI Detection Solution
Choosing the appropriate AI detection tool is critical.
Organizations should look for solutions that meet their industry needs and comply with relevant regulations.
For example, in healthcare, tools must adhere to HIPAA requirements to protect patient data.
Regular updates and robust customer support are also essential when selecting a tool.
Establishing Policies for Responsible AI Use
Clear organizational policies are vital for guiding the use of AI detection tools.
These policies should outline acceptable AI practices, procedures for detecting AI-generated content, and guidelines for handling flagged material.
By setting these standards, organizations can ensure ethical and compliant AI usage.
Training Teams on AI Detection Capabilities
Educating employees about AI detection tools and their capabilities is key to successful integration.
Training should focus on how the tools work, their limitations, and the importance of human oversight.
This empowers teams to use detection tools effectively and understand their role in maintaining content authenticity and compliance.
Updating Systems to Address Evolving AI Models
AI models are continually evolving, and updating detection systems regularly is crucial.
These updates ensure tools remain effective in identifying increasingly sophisticated AI-generated content.
Monitoring advancements in AI technology helps organizations stay ahead and adapt their detection strategies as needed.
Combining AI Tools with Human Oversight
While AI detection tools provide valuable automation, human oversight is essential for accuracy.
Human reviewers can validate flagged content, provide contextual understanding, and address cases where detection tools may fall short.
This combination of machine efficiency and human judgment ensures reliable and trustworthy results.
Boost Your Productivity With Knapsack
AI detection is becoming an essential part of maintaining data integrity, ensuring compliance, and protecting sensitive information across industries.
Integrating the right tools into your professional workflow can streamline your operations and enhance your organization's security and efficiency.
Knapsack offers innovative solutions tailored to your needs, helping you optimize AI usage while maintaining privacy and trust.
Visit Knapsack today to explore how our platform can empower your team and boost productivity in a secure and efficient way.