What’s the Role of Smaller AI Models in Mobile Computing?
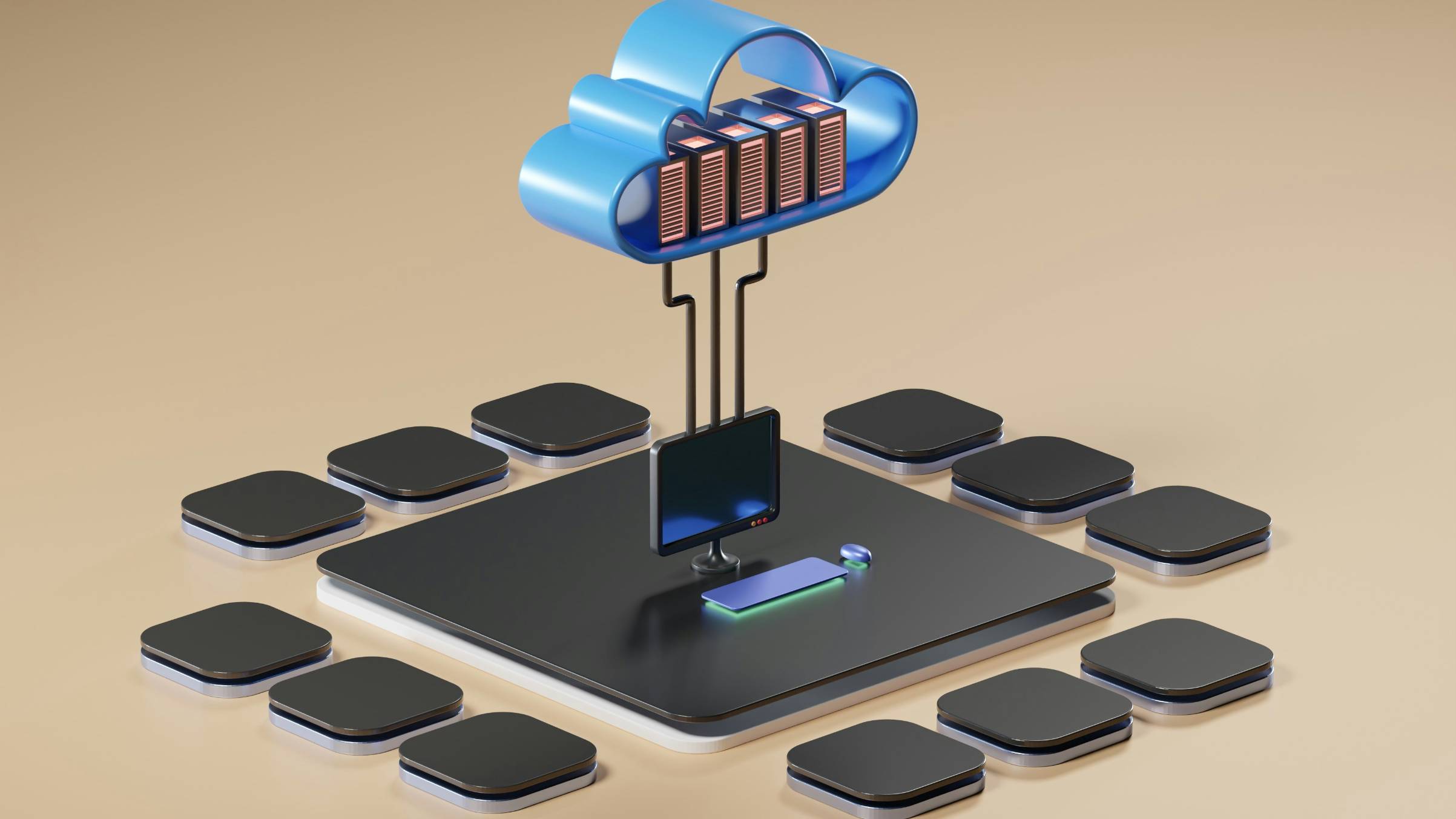
Mobile computing is rapidly evolving, with artificial intelligence (AI) playing a crucial role in enhancing the capabilities of smartphones, tablets, and wearable devices. Traditionally, AI models have been large and resource-intensive, making them unsuitable for mobile platforms with limited processing power and battery life.
However, a shift towards smaller AI models is now redefining how AI operates in mobile environments. These lightweight models offer faster processing times, reduced energy consumption, and improved privacy, making them ideal for real-time applications and on-device computing.
The rise of smaller AI models is not just a trend, but a transformation in how we engage with mobile technology. These models bring the power of AI to our fingertips, without compromising on performance or user experience. As mobile applications grow in complexity, the need for efficient, resource-light AI solutions becomes more than just a requirement-it's a pressing necessity that is shaping the future of mobile computing.
Benefits of Smaller AI Models in Mobile Computing
As mobile devices become more powerful and integral to daily life, the demand for AI on mobile platforms has increased dramatically. Smaller AI models offer a solution to some of the significant limitations of mobile computing, providing benefits from efficiency to privacy.
Increased Efficiency and Speed
Smaller AI models are designed to run more efficiently on mobile devices with limited processing power and memory. These models can reduce inference latency by up to 40%, enabling faster responses in real-time applications such as virtual assistants, mobile games, and augmented reality. By running efficiently on-device, they avoid the delays associated with cloud-based AI processing, improving the overall user experience.
Cost-Effectiveness
Smaller AI models enhance performance and significantly reduce operational costs. By requiring fewer computational resources, these models enable businesses to run AI-driven applications on mobile platforms without expensive cloud infrastructure. Smaller models can reduce operational costs by 30-40%, making AI more accessible for businesses of all sizes, from startups to large enterprises. This democratization of AI allows for broader adoption of advanced technologies on mobile devices.
Energy Efficiency and Sustainability
Energy consumption is a significant concern in mobile computing, as power-intensive tasks can drain battery life quickly. Smaller AI models consume 20-30% less energy than their larger counterparts, extending battery life and making mobile devices more sustainable. This is especially important for applications that require continuous processing, such as health monitoring apps or real-time navigation, where energy efficiency is critical for user satisfaction.
Enhancing Data Privacy with On-Device AI
As data privacy and security concerns continue to rise, especially in mobile applications, smaller AI models play a crucial role in addressing these issues. These models offer significant privacy advantages by processing data locally on the device, allowing sensitive information to stay on the user’s mobile device rather than being sent to cloud servers for processing.
Local Data Processing
One of the primary benefits of smaller AI models is their ability to process data directly on the device. This approach enhances privacy by ensuring that personal information, such as location data, health metrics, or financial transactions, remains on the user’s mobile device. By avoiding transmitting sensitive data to cloud servers, smaller AI models can improve data privacy by up to 25%, which is especially important in industries like healthcare, where strict privacy regulations are mandatory.
Regulatory Benefits
With stricter data privacy regulations like GDPR and HIPAA in place, mobile applications must ensure that they handle user data securely and compliantly. Smaller AI models enable on-device processing, reducing the need to transfer data across networks and thereby minimizing the risk of breaches or unauthorized access. This capability is precious in industries where the handling of sensitive personal information is heavily regulated, such as finance and healthcare.
Applications of Smaller AI Models in Mobile Computing
Smaller AI models are rapidly gaining traction across various industries as their efficiency, speed, and ability to process data locally make them ideal for mobile applications. Here are a few key areas where these models are making an impact:
Mobile Personal Assistants
Voice-activated personal assistants, such as those in smartphones, increasingly rely on smaller AI models to provide faster, more responsive services. These models are used for tasks like real-time language processing, voice recognition, and personalization based on user preferences. The efficiency of smaller models ensures that these assistants can deliver results quickly without draining battery life or relying heavily on cloud computing, which could compromise privacy.
Healthcare
In healthcare, smaller AI models power mobile health apps for diagnostics, monitoring, and real-time insights. These models can analyze patient data directly on the mobile device, offering fast and actionable feedback to patients and healthcare providers. This real-time analysis helps improve patient outcomes while maintaining high levels of privacy, as sensitive health data is processed locally, avoiding cloud-based risks.
Mobile Gaming and Augmented Reality
Smaller AI models transform mobile gaming and augmented reality (AR) experiences by delivering faster, more responsive interactions. These models can process visual and sensory data in real time, allowing mobile games and AR applications to provide immersive and smooth experiences. The low latency smaller models offer makes them ideal for applications requiring real-time feedback and interactions.
Challenges in Deploying Smaller AI Models on Mobile Devices
Despite the benefits of smaller AI models, their deployment on mobile devices comes with challenges. These hurdles must be addressed to fully unlock their potential in mobile computing.
Model Optimization and Accuracy
One of the main challenges in using smaller AI models is maintaining a balance between model size and accuracy. As models are downsized to run efficiently on mobile devices, there is a risk of losing some functionality and precision. While smaller models are designed to be resource-efficient, developers must ensure they retain enough complexity to deliver accurate results, especially in applications like healthcare or financial transactions, where precision is critical. Striking this balance requires careful model optimization, which can be time-consuming and complex.
Hardware Limitations
Although mobile hardware continues to improve, there are still limitations regarding processing power, memory, and battery life. Running AI models on mobile devices, even smaller ones, can strain these resources. This is especially true for more advanced applications, such as augmented reality or high-resolution image recognition, which require significant computational power. As AI models evolve, mobile hardware must keep pace to ensure smooth performance without compromising the user experience.
Boost Your Mobile AI Capabilities with Knapsack
For developers and businesses looking to integrate efficient, privacy-focused AI into mobile applications, Knapsack offers the ideal solution. Our platform supports deploying smaller AI models ideally suited for mobile environments. These models provide fast, real-time processing while minimizing resource consumption and ensuring data privacy by keeping computations on-device. Whether enhancing mobile personal assistants, healthcare apps, or mobile gaming, Knapsack can help you implement AI solutions that deliver performance and scalability.
Visit Knapsack today to explore how our AI solutions can transform your mobile computing applications.